Generative AI combined with bioinformatics: a wide range of applications
What happens when generative AI is trained on scientific texts from the biological literature, databases, genetic sequences or code? Explore its applications led by our scientists to boost research, to improve and innovate on tools, and accelerate discovery in different life science domains. These new technologies also come with challenges and shortcomings. Learn how SIB experts tackle them.
Examples in the medical field:
Of generative AI and LLMs
Generative Artificial Intelligence (AI) encompasses systems capable of creating new content, from text and images to videos, music and much more. Large Language Models (LLMs), a key type of Generative AI, are trained on extensive text data, including genetic sequences or informatic code, to summarize, generate and predict new content. Models such as ChatGPT and BioBERT exemplify this, with ChatGPT excelling in generating text for chatbots and creative writing, while BioBERT focuses on (i.e. is pre-trained on) biomedical text. LLMs employ deep- learning techniques, particularly transformers, to analyse and understand language patterns from vast datasets, and to predict the next ‘word’ or sequence of words based on context.
Fast generation of custom antibodies to fight disease
Monoclonal antibodies are special proteins produced in the laboratory. By cloning a single type of immune cell, it is possible to obtain a large quantity of identical antibodies that can recognize and bind to their target with high precision. These targets include, for instance, germs or diseased cells. However, their traditional discovery is very time-consuming. The SIB Group of Andrea Cavalli is working on AntibodyGPT, a language model to predict the chemical structure of an antibody with a desired property, to accelerate their development.
Of generative AI and LLMs
Generative Artificial Intelligence (AI) encompasses systems capable of creating new content, from text and images to videos, music and much more. Large Language Models (LLMs), a key type of Generative AI, are trained on extensive text data, including genetic sequences or informatic code, to summarize, generate and predict new content. Models such as ChatGPT and BioBERT exemplify this, with ChatGPT excelling in generating text for chatbots and creative writing, while BioBERT focuses on (i.e. is pre-trained on) biomedical text. LLMs employ deep- learning techniques, particularly transformers, to analyse and understand language patterns from vast datasets, and to predict the next ‘word’ or sequence of words based on context.
Answering medical questions in radiation oncology
In an exploratory study involving the SIB Group of Janna Hastings, the remarkable ability of ChatGPT to answer questions in the medical field was tested in the specialized case of radiation therapy. It responded accurately to most multiple-choice questions (94%), but less so for open-ended responses, as assessed by oncologists (48%). Such inconsistency makes such models unsuited as a self-contained source of medical information, but their language capabilities make them an exciting new user interface for databases and guidelines.
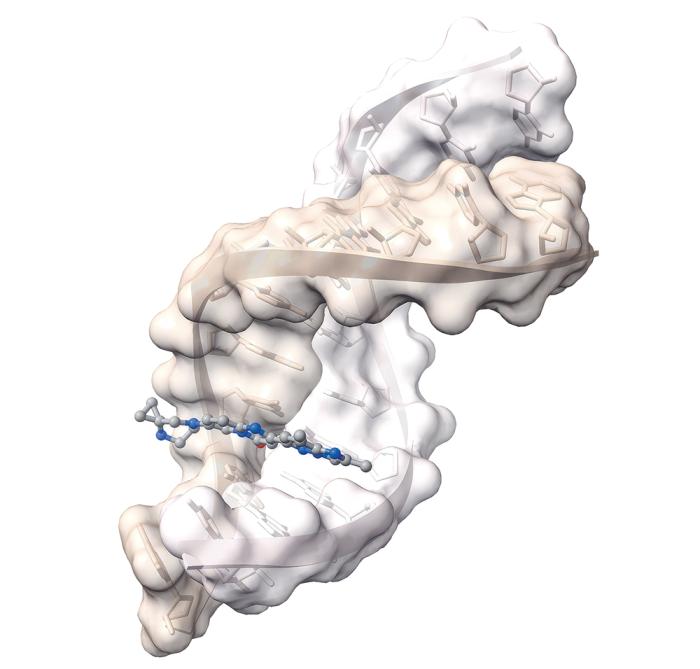
Deciphering the hidden role of RNA in cancer
The SIB group of Raphaëlle Luisier is teaming up with experts in Natural Language Processing at SIB and IDIAP to study RNA, molecules which carry genetic instructions and help make proteins in living cells. They are interested in parts of RNA that do not directly code for proteins, and how they affect complex human disorders, such as neurodegeneration and cancer. In melanoma, a type of skin cancer, some treatments do not work well over time, especially drugs called BRAF inhibitors, and RNA could play a role.
Examples in the biological field:
Understanding how insects shed their skin
Arthropods, such as insects and spiders, are Earth’s most diverse creatures, vital for nature, farming and health. The periodic shedding of their outer shell, called moulting, is key to their adaptability. However, to study this process, an integrated reference for arthropod naming is lacking. As part of a Sinergia collaboration, the SIB Groups of Marc Robinson-Rechavi and Frédéric Bastian and of Robert Waterhouse integrated species name data with sequence data from different public databases using generative AI methods into the MoultDB resource, serving as a reference for the field.
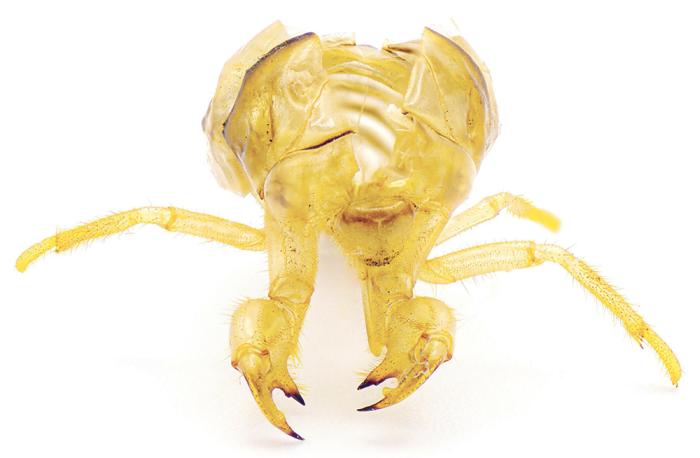
Conversing with complex biological databases
Can ChatGPT-like technologies support life science researchers in exploring data they are not familiar with? This is the question our new Knowledge Representation unit investigated, through concrete examples from SIB’s leading open databases and software tools. They showed the potential of conversational AI to describe biological datasets, as well as generate and explain complex queries across them. While the benefits include leveraging the wealth of open data, authors also stressed that caution should be exercised in the process.
Read the news "Bringing meaning to biological data: knowledge graphs meet ChatGPT"
Generative AI and biocuration: a virtuous cycle
The interplay between the possibilities offered by AI, and LLMs in particular, and the importance of human expertise is well illustrated in the context of biocuration, where SIB is a recognized leader. Biocuration is the art of expertly extracting knowledge from the biological and biomedical literature to build an accurate, reliable and up-to-date encyclopedia serving science at large.
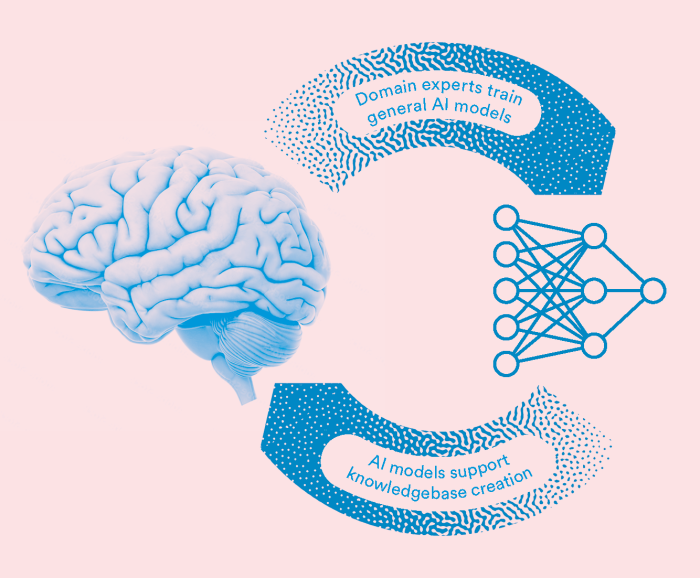
Predicting protein structure, function and sequence thanks to high-quality data
The function of a protein is a pivotal piece of information to understand molecular processes involved in disease, drug development or enzymatic activity. This function results from the protein’s 3D structure, itself determined by its sequence of amino acids. Today, generative AI models can be used to predict:
- A protein structure from its sequence, which could be used to design new drugs that bind it.
- A protein function from its sequence, which could help to annotate a newly assembled genome, the blueprint for life.
- A protein sequence that could carry out a specific function, such as degrading an environmental pollutant.
For this, many models, from Google DeepMind’s AlphaFold to ProtGPT2, are trained on the universal protein knowledgebase UniProt, co-developed by SIB, and where proteins are extensively and reliably curated.
Read the news "Artificial intelligence tools shed light on millions of proteins"