ATGCCGGAATTGGCACATAACAAGTACTGCCTCGGTCCTTAAGCTGTATTGCACCATATGACGGATGCCGGAATTGGCACATAACAAGTAC
TGCCTCGGTCCTTAAGCTGTATTGCACCATATGACGGATGCCGGAATTGGCACATAACAACGGTCCTTAAGCTGTATTGCACCATATGACG
GATGCCGGAATTGGCACATAACAAGTACTGCCTCGGTCCTTAAGCTGTATTTCGGTCCTTAAGCTGTATTCCTTAACAACGGTCCTTAAGG
ATGCCGGAATTGGCACATAACAAGTACTGCCTCGGTCCTTAAGCTGTATTGCACCATATGACGGATGCCGGAATTGGCACATAACAAGTAC
TGCCTCGGTCCTTAAGCTGTATTGCACCATATGACGGATGCCGGAATTGGCACATAACAACGGTCCTTAAGCTGTATTGCACCATATGACG
GATGCCGGAATTGGCACATAACAAGTACTGCCTCGGTCCTTAAGCTGTATTTCGGTCCTTAAGCTGTATTCCTTAACAACGGTCCTTAAGG
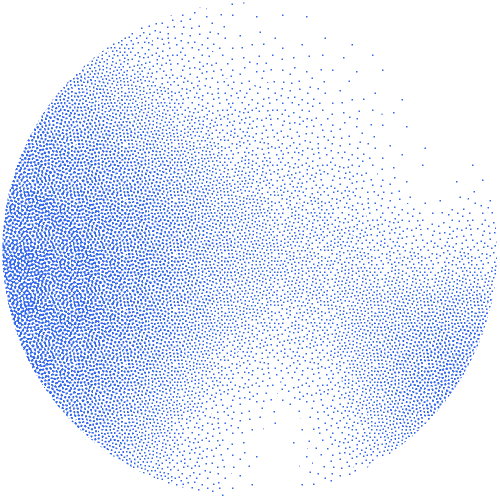
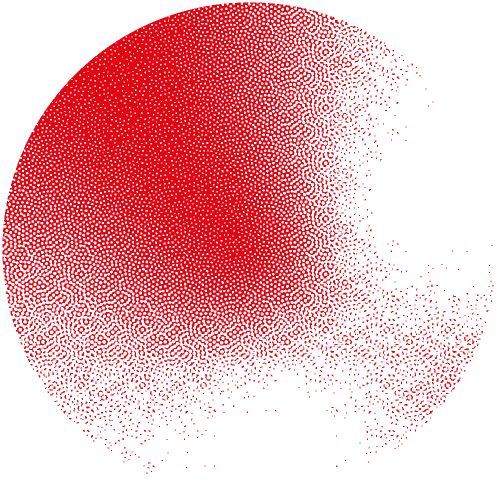
The medical knowledge and decision support group aims to develop and evaluate novel informatics approaches for biomedical knowledge discovery, personalised health, and clinical data management and decision support.
Methodologically, we develop novel artificial intelligence approaches that combine prior knowledge, often formally represented as ontologies, with large-scale machine learning from data, in order to make knowledge-informed predictions. We also develop and evaluate approaches for enhanced interpretability of predictive models and evaluate these with users and clinicians in order to explore experiences with, and trust in, artificial intelligence as mediated by different forms of visualisation and explanation.
Our main topic areas of expertise are metabolic health and mental health. Activity monitoring with feedback, for example through wearable activity sensors, has already emerged as a prominent strategy to digitally support healthy behaviours and improve metabolic health. Our group aims to develop methods that are able to integrated information from individual clinical history, temporal metabolic state and activity sensors using artificial intelligence algorithms to provide individualised feedback on metabolic health and support early detection of biomarkers of metabolic dysregulation. We also apply these strategies to help interpret and understand mental health from a patient-centred perspective supported by digital experiential data capture and interpretation.
One of our core areas of interest is the development of approaches that aim to advance knowledge-driven partial automation of biomedical evidence synthesis and thereby to accelerate the translation of evidence intoimplementation.